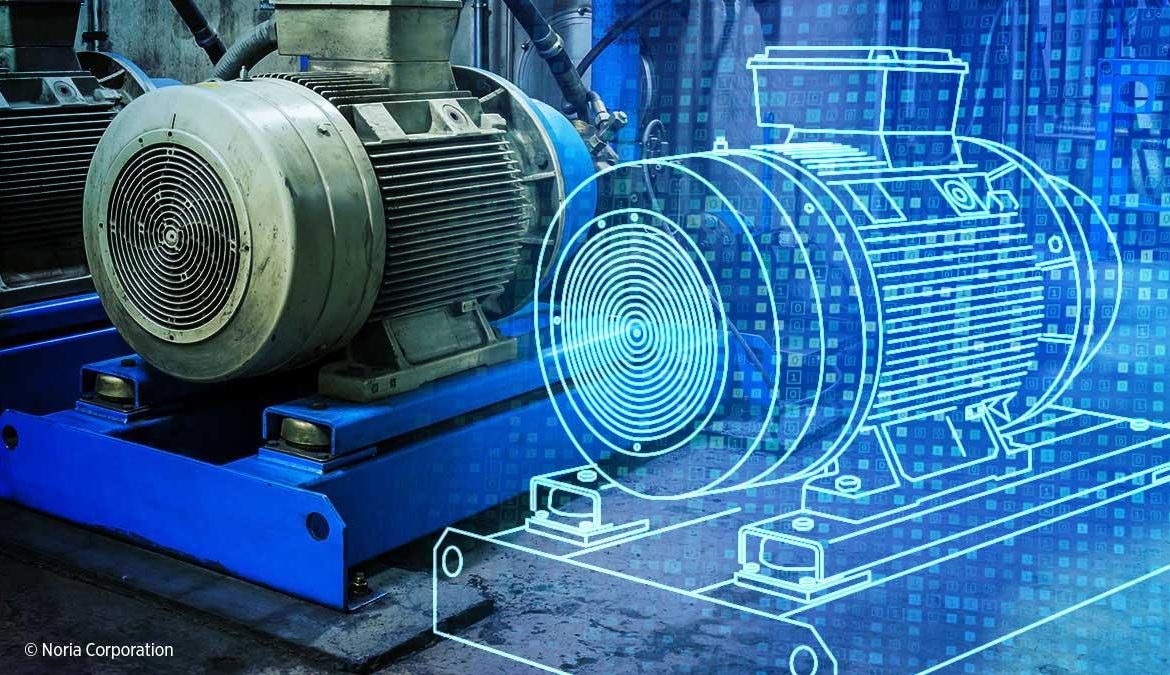
Predictive maintenance (PdM) is a technique that uses data analytics and machine learning to foresee problems with equipment before they occur. The PdM allows companies to take corrective action before a failure happens, resulting in increased uptime and reduced costs in production.
There is no question that predictive maintenance can be highly effective, but there are also some common challenges that companies face when implementing the PdM program. This article will discuss some of the most common problems with predictive maintenance and how to avoid them.
The top 4 challenges of predictive maintenance
1. Lack of data
Predictive maintenance requires large amounts of data. To predict equipment problems, you need real-time data about that equipment. This data can come from various sources, including sensors, control systems, and maintenance records.
However, many companies do not have complete data sets for their equipment, making it challenging to develop an effective predictive maintenance program.
There are a few ways to overcome this problem. Option include:
Work with the limited data available. Even if you don’t have complete data sets, you may be able to use statistical methods to develop predictions.
Support your data with data from similar equipment. This can be helpful if you have limited data for the specific type of equipment you’re trying to predict problems for.
Use data from other sources to develop predictions. For example, if you’re trying to predict problems with a particular type of machine, you could look at data from similar systems in other industries.
The above solutions may give you some insight to foresee the developing problems but do not forget that every piece of equipment has unique characteristics and a working environment that requires individually monitored for fault predictions.
2. Implementation
It is essential to collect data in a way that allows PdM platforms to access and analyze it, leveraging the power of AI to convert the raw data into actionable insights. The solution to obtaining a constant stream of relevant data is smart sensors or data acquisition systems with machine learning algorithms.
Artesis does not require any difficult wiring and assembly processes.
Smart sensors are connected to the internet and can provide the real-time data companies require for PdM. Additionally, condition monitoring systems such as vibration analysis can detect equipment problems before they lead to failures. Depending on the environment, installing sensors or collecting them without electromagnetic noises can be difficult or even impossible such as in submersible pumps or steel industries.
Artesis predictive maintenance solutions do not require sensors. It is sufficient to install the system in the control cabinet of the rotating machine. This eliminates the need for a difficult and costly implementation process.
3. Data Security
Another common challenge of predictive maintenance is data security. Since predictive maintenance systems rely on data, companies must ensure that this data is secure.
Data security is challenging because it’s often difficult to control access to the data flow due to its volume and complexity. Additionally, companies need to be careful about where they store their data. Storing data in the cloud can be convenient, but it also comes with security risks.
There are a few ways to overcome this challenge:
- Restrict access to data. Ensure only authorized person has access to the data used for predictive maintenance.
- Encrypt data. Encryption ensures that data is not accessible if it falls into the wrong hands.
- Store data securely. Keep data in a secure location, such as a private cloud or on-premises server.
4. Building a team of data analysts
For PdM to be effective, it is necessary to have a talented team of data analysts. This team will be responsible for developing the predictions at the heart of PdM programs.
Data analysts need to understand data analytics and machine learning deeply. They should also be familiar with the specific type of equipment that they’re working with.
Predictive maintenance relies on data analytics and machine learning to function effectively. It requires training and expertise to set up and maintain. Not all companies have the internal resources to develop predictive maintenance programs, which presents a barrier to implementation.
There are a few ways to overcome this challenge:
- Hire data analysts with the necessary skills. Successful recruitment may require looking outside of your traditional hiring pool.
- Train existing employees. If you have employees interested in learning data analytics and machine learning, you could train them to work on your predictive maintenance team.
- Outsource the development of your predictive maintenance program. If you don’t have the internal resources to develop a predictive maintenance program, you could consider outsourcing this task to a company specializing in these programs.
Conclusion
Artesis predictive maintenance solutions;
- Artesis offers innovative predictive maintenance solutions to overcome all these challenges.
- Sensorless predictive maintenance technology eliminates the hassle of accessing equipment.
- Access data from anywhere thanks to cloud-based monitoring technology.
- Effective in detecting both mechanical and electrical faults.
- Data collection is simple and efficient. Artesis’ unique know-how makes it easy for you to detect malfunctions in rotating equipment.
- It does not require expertise, it is very easy and practical to use and install.