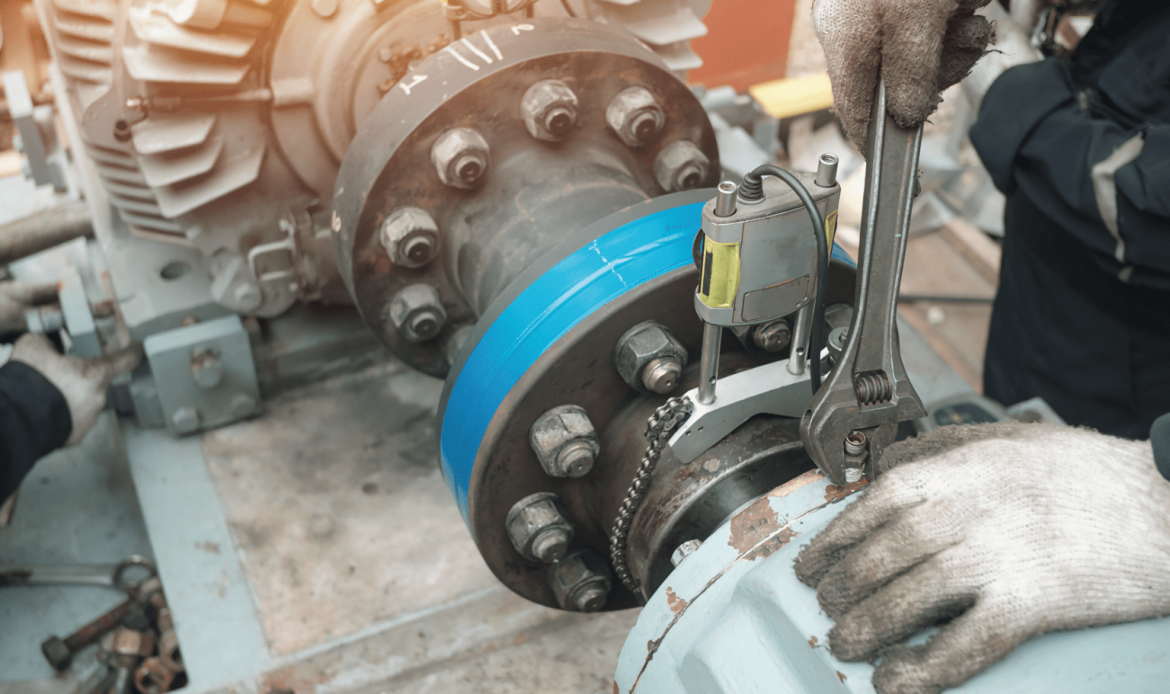
Predictive maintenance is a technique that uses data and analytics to predict when equipment is likely to fail, allowing maintenance to be scheduled in advance and improving overall reliability. In the coming years, advances in technology and the increasing availability of data are likely to drive significant changes in how predictive maintenance is used.
In this article, we will explore some key trends in predictive maintenance that will likely shape the industry in 2023 and beyond.
Key Predictive Maintenance Trends for 2023 and Beyond
Below is a list of the 7 top predictive maintenance trends expected for the coming year.
Artificial Intelligence
The use of artificial intelligence and machine learning will become more widespread. These technologies allow for the analysis of large amounts of data in real-time, making it possible to identify patterns and trends that can be used to predict when equipment is likely to fail. This allows for more accurate and timely maintenance, improving overall reliability and reducing downtime.
Internet of Things
The Internet of Things (IoT) will play a more prominent role in predictive maintenance. IoT devices, such as sensors and smart meters, can collect real-time data on equipment performance. This information can then be used to identify potential problems and schedule maintenance before failures occur.
Inspection Technologies
Predictive maintenanceinvolves the use of systematic condition monitoring to prevent equipment failures. This can be achieved through the use of inspection technologies, such as infrared thermography, ultrasonic analysis, vibration monitoring, and robotic inspection. These techniques are non-invasive and enable precise and cost-effective testing of equipment. Combining multiple methods makes it possible to accurately determine the source of degradation and improve asset reliability.
Digital Twins
The current state of technology is not advanced enough to accurately track the dynamic changes that occur in manufacturing operations and equipment. Digital twins offer a solution by creating a virtual replica of physical equipment, allowing manufacturers to continuously monitor its actual working conditions and visualize future scenarios. Manufacturers can predict anomalies and problematic areas that could cause equipment failure by using digital twins in predictive maintenance. Additionally, digital twins can simulate different situations to understand the risk factors that affect machine performance, leading to more informed decision-making and actionable plans to prevent critical problems.
Predictive Analytics
Predictive maintenance solutions that use predictive analytics can analyze unstructured data, such as sensor readings, past reports, and environmental parameters, to predict equipment failures. To support this, startups and scaleups are developing analytical tools to synthesize and cluster data. Big data platforms can also improve the transparency of system health conditions and enable precise modeling and effective forecasting by examining patterns in large datasets. The data-driven predictions provided by these solutions can offer valuable insights for better-informed business decisions and reduced turnaround time.
Predictive Maintenance as a Service
Predictive maintenance as a service (PdMaaS) is making predictive maintenance more accessible and affordable for manufacturing plants. With this, startups are offering cloud-based PdMaaS solutions that can reduce infrastructure costs and allow manufacturers to leverage existing components. These solutions also enable on-demand access to predictive maintenance and improve scalability and remove the need for infrastructure and development costs.
Immersive Technologies
Startups are using extended reality (XR) technologies, such as augmented reality (AR) and virtual reality (VR), to simplify asset inspections. These platforms make it easier to perform on-site inspections, document failures, and follow up on paperwork. Using headsets and smart glasses, technicians can visualize historical data about components, speeding up in-situ diagnostics. At the same time, AR can collect data from equipment sensors to support predictive maintenance planning. Overall, immersive technologies like AR and VR can enhance on-the-spot visualization and enable early fault detection.
Final Thoughts
In the coming years, technological advances and data availability will drive changes in predictive maintenance. Key trends include using AI and machine learning, the integration of IoT, cloud computing, inspection technologies, and predictive maintenance as a service. By leveraging these technologies and data, businesses can stay ahead of potential problems and keep equipment running smoothly.